Real data reconstruction#
This tutorial covers real data reconstruction using ToMoBAR software. The data is obtained at Diamond Light Source facility (UK synchrotron), i12 beamline. The sample is a magnesium allow which undergoes some thermal changes in which the dendritic growth occurs. See more details about the experiment in [GUO2018] and more on reconstruction using ToMoBAR in [KAZ2017].
This tutorial loosely follows Demo_RealData.py demo.
We will extract a 2D sinogram out of 3D projection data and reconstruct it using the FBP method.
from tomobar.methodsDIR import RecToolsDIR
Rectools = RecToolsDIR(
DetectorsDimH=detectorHoriz, # Horizontal detector dimension
DetectorsDimV=None, # Vertical detector dimension
CenterRotOffset=None, # Center of Rotation scalar
AnglesVec=angles_rad, # A vector of projection angles in radians
ObjSize=N_size, # Reconstructed object dimensions (scalar)
device_projector="gpu",
)
FBPrec = Rectools.FBP(sinogram, data_axes_labels_order=["detX", "angles"])
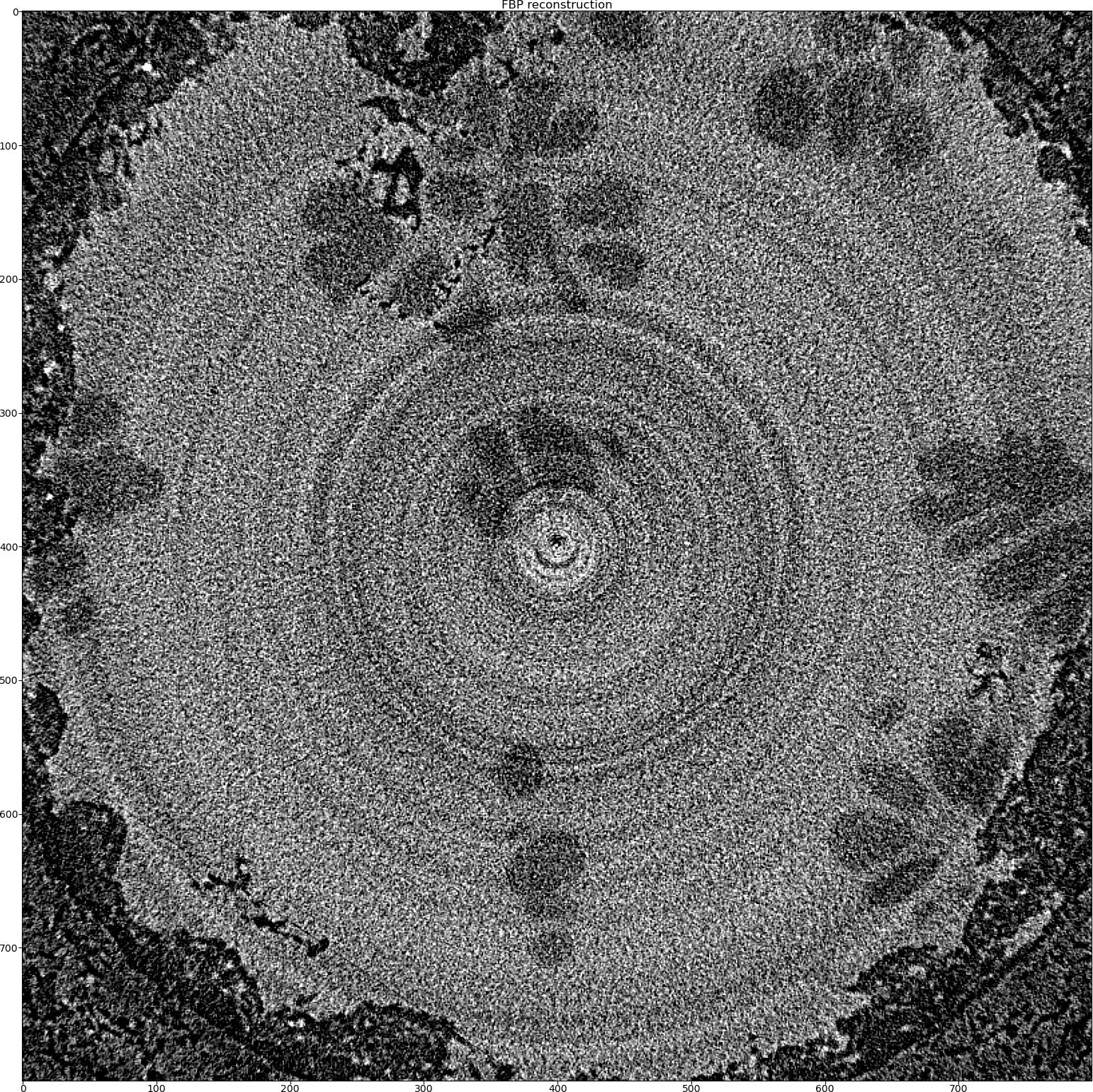
Next we reconstruct using ordered-subsets FISTA with Total Variation regularisation.
from tomobar.methodsIR import RecToolsIR
Rectools = RecToolsIR(
DetectorsDimH=detectorHoriz, # Horizontal detector dimension
DetectorsDimV=None, # Vertical detector dimension (3D case)
CenterRotOffset=None, # Center of Rotation scalar
AnglesVec=angles_rad, # A vector of projection angles in radians
ObjSize=N_size, # Reconstructed object dimensions (scalar)
datafidelity="PWLS", # Data fidelity term
device_projector="gpu",
)
_data_ = {
"projection_norm_data": sinogram, # Normalised projection data
"projection_raw_data": sinogram_raw, # Raw projection data
"OS_number": 6, # The number of subsets
"data_axes_labels_order": ["detX", "angles"],
}
lc = Rectools.powermethod(_data_) # calculate Lipschitz constant
_algorithm_ = {"iterations": 25, "lipschitz_const": lc}
_regularisation_ = {
"method": "PD_TV", # Regularisation method
"regul_param": 0.000002, # Regularisation parameter
"iterations": 60, # The number of regularisation iterations
"device_regulariser": "gpu",
}
RecFISTA = Rectools.FISTA(_data_, _algorithm_, _regularisation_)
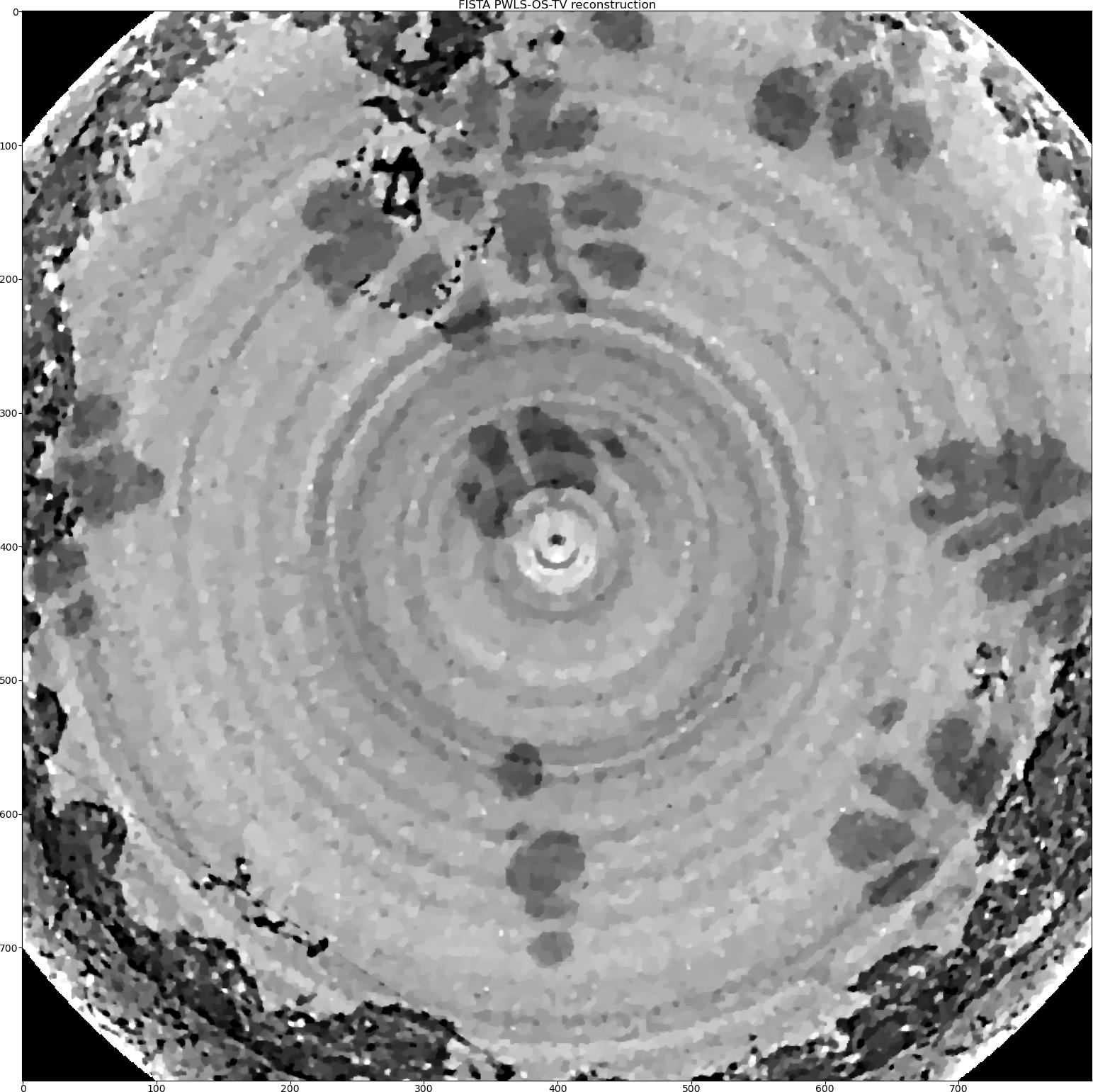
Then we will add the Group-Huber data fidelity model [PM2015] to minimise the ring artefacts. The only change needed is the update of the _data_ dictionary.
_data_.update({"ringGH_lambda": 0.000015})
_data_.update({"ringGH_accelerate": 6})
RecFISTA = Rectools.FISTA(_data_, _algorithm_, _regularisation_)
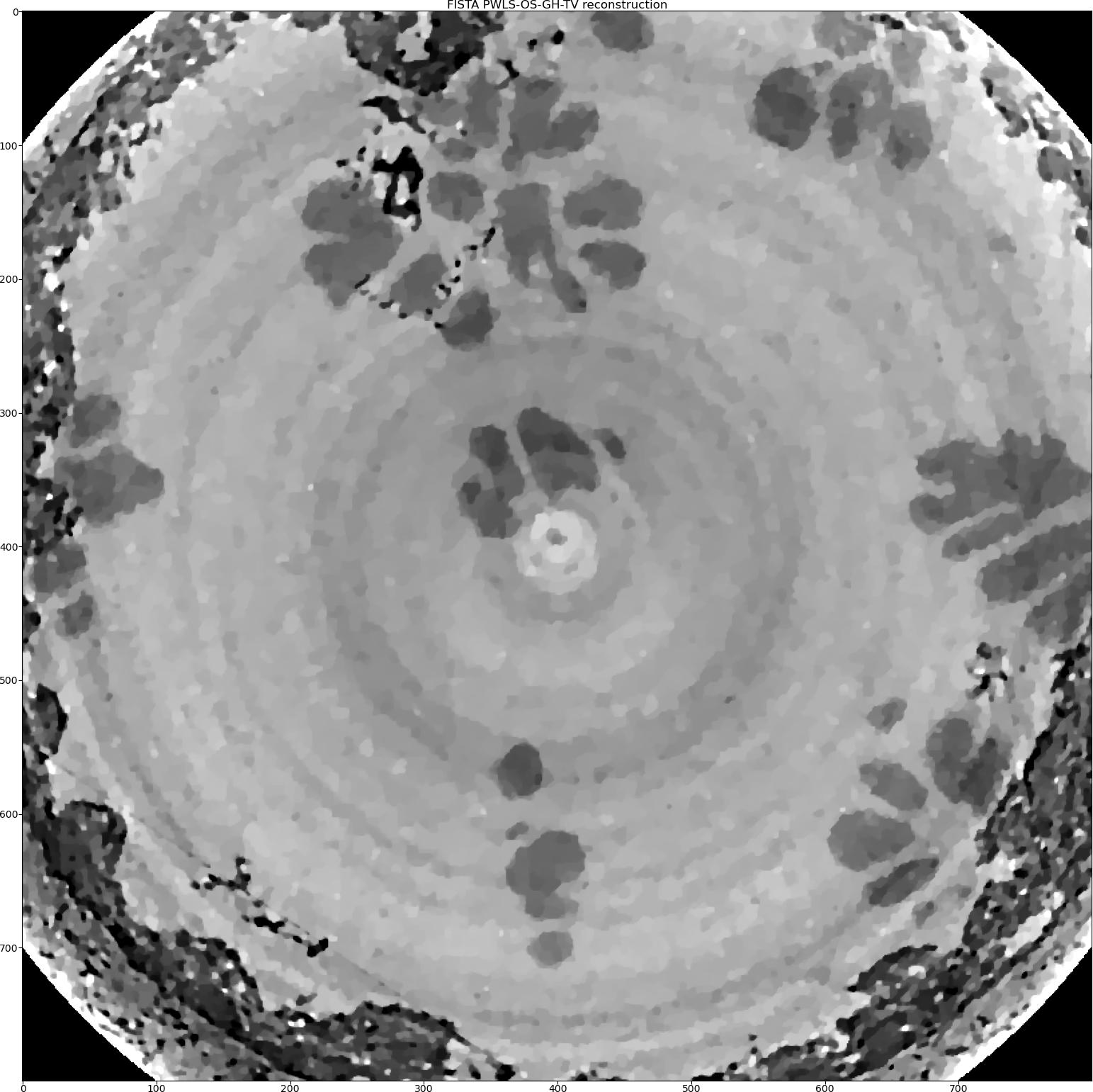
We also can try the Stripe-Weighted Least Squares (SWLS) data model [HOA2017]. As we change the data fidelity, we need to re-initialise the class object.
Rectools = RecToolsIR(
DetectorsDimH=detectorHoriz, # Horizontal detector dimension
DetectorsDimV=None, # Vertical detector dimension (3D case)
CenterRotOffset=None, # Center of Rotation scalar
AnglesVec=angles_rad, # A vector of projection angles in radians
ObjSize=N_size, # Reconstructed object dimensions (scalar)
datafidelity="SWLS", # Data fidelity term
device_projector="gpu",
)
_data_ = {
"projection_norm_data": sinogram, # Normalised projection data
"projection_raw_data": sinogram_raw, # Raw projection data
"OS_number": 6, # The number of subsets
"beta_SWLS": 0.2, # parameter for the SWLS model
"data_axes_labels_order": ["detX", "angles"],
}
RecFISTA = Rectools.FISTA(_data_, _algorithm_, _regularisation_)
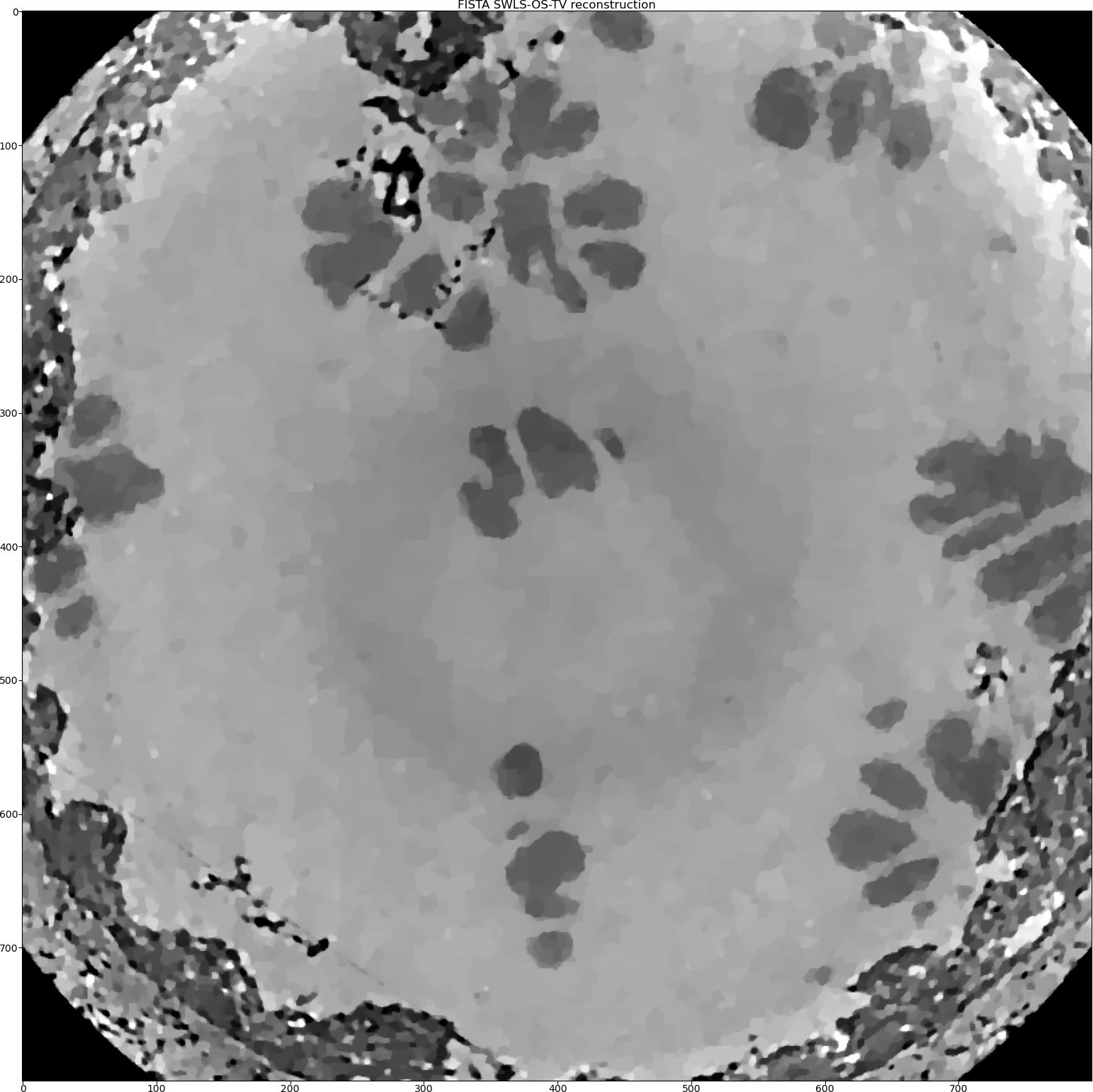
As one can see that visually the SWLS model produced the best reconstruction here. This model is indeed works very well when the stripes (rings) are full and not partial.